The IMD Wrap: Talkin’ ’bout my generation
As a Gen-Xer, Max tells GenAI to get off his lawn—after it's mowed it, watered it and trimmed the shrubs so he can sit back and enjoy it.
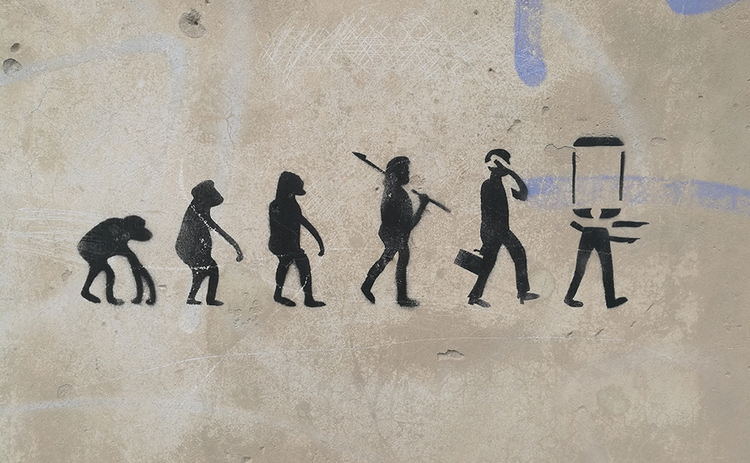
I’m a member of Generation X, so the kids these days tell me: I was born in the 1970s, I’m grumpy, scared of change, and my biggest tech achievement is learning to work “The Facebook.” Then, there’s Gen Y, also known as Millennials, who have time each morning to turn up their pant legs just so and oil their beards perfectly, yet apparently not enough time to make it into the office before 10 am. Then there’s Gen Z, and finally GenAI, the laziest generation of all, who expect to ask a question and get all the answers immediately, without having to do any research of their own, for the mere price of selling their soul to Skynet or The Matrix (each a masterpiece in its own right, created by—and exploiting the paranoia of—different generations) or another doomsday scenario.
Wait, that’s not what GenAI means? OK, so let’s take a closer look.
There’s a confusing number of types of artificial intelligences in use, from machine learning and natural-language processing to deep learning, neural networks, and swarm intelligence—so many that tech observers can’t even consistently keep track of how many there are and what they should be called. And being a Gen-Xer, I don’t understand any of them beyond my irrational fear that computers will take over my job. That’s also why I unplug my toaster every night, so it can’t kill me in my sleep.
But it’s the relatively recent work on generative AI conducted by the Microsoft-backed OpenAI initiative that has captured peoples’ and corporations’ imaginations, and set the industry abuzz with ChatGPT-like tools, while raising fears among regulators in Europe and the US. And it’s not just big tech firms riding the AI wave, like Google, IBM, and AWS; Bloomberg rolled out its own tool, BloombergGPT, to improve some of the charting and search features of its terminal, while S&P Global is developing ChatIQ, a conversational interface that will provide results to users’ queries by combining information from across the data giant’s vast wealth of databases. And financial firms such as HSBC, BNY Mellon and Brown Brothers Harriman, among others, are using AI to power a broad range of functions, including risk and AML.
So, just what is GenAI? And why are people so excited and worried about it? Any kind of AI can be trained to find and present content, or to automate repetitive functions, or to provide canned responses to simple questions (think customer service chatbots), or help you write a document or text message (think about the predictive text functions in the latest version of Microsoft Word, or the predictive text and autocorrect function on your mobile phone). The difference with GenAI is its creative element—the power to not only collect information, sift through it, identify what’s relevant and return it as the result of a search query, but to organize that information into an easily readable, free-text format, rather than a bunch of links or numerical tables.
I’m so looking forward to see what’s coming because I don’t think it’s going to replace people writing code; it’s going to augment their roles. It will make things quicker.
Linda Powell, BNY Mellon
In the world of finance, this has the potential to drastically shorten timeframes for performing manual research tasks, and to take over functions that have traditionally been manual and labor-intensive, but which can be largely automated. This doesn’t mean that workforces will be fired and replaced wholesale, but it does mean that those workforces can now do their jobs more efficiently and focus their efforts on more time-consuming aspects that still require a human touch.
This is pretty much what Linda Powell, enterprise head of data governance and deputy CDO at BNY Mellon, said in a presentation at last week’s Operational Efficiency Summit, hosted in New York by WatersTechnology and our sibling brands Risk.net and Chartis Research.
“I’m so looking forward to see what’s coming because I don’t think it’s going to replace people writing code; it’s going to augment their roles. It will make things quicker,” Powell said, speaking about the new wave of technology evolution, including AI, and how it can benefit—and also depends on—good data quality and metadata.
“I used to write code, and I used to hate writing code because I’d always put the commas in the wrong place,” she confessed. “Now there are apps that I can point at a dataset and say, ‘Profile that.’ In the past, I would need to write the code to do that. Now, it’s so much faster. And now, the apps can even say, ‘I suggest this, and if you accept my suggestion, I’ll write the code to do it.’”
And while the ability to get the most out of AI hinges on accurate data and good metadata, it can also contribute to improving data quality. For example, Powell gave the example of how AI can help figure out whether an individual or company with the same name across multiple client databases is the same person or multiple people. BNY uses—and invested in—Quantexa, a New York-based “decision intelligence platform” provider that also counts HSBC, Standard Chartered, and ING among its customers, to support functions including data management, customer intelligence, risk management, fraud detection, AML and KYC.
By using Quantexa’s platform, instead of needing an analyst to investigate each single instance of the same name, Quantexa can analyze the profiles to state with a degree of confidence which profiles are the same person or duplicates, and which are separate individuals, reducing the number of in-depth investigation that an analyst needs to do.
The bottom line: Leveraging new technologies helps you make better decisions, faster. It doesn’t necessarily make you redundant.
If, that is, you (a) have the data, and (b) have meticulously tagged it with consistent metadata standards. Bloomberg and S&P both spent considerable effort doing this—S&P, for example, leveraging its $550 million acquisition of AI startup Kensho in 2018, a price tag that seemed shocking at the time but is already paying dividends.
But AI isn’t just within the reach of the largest and best-funded companies. In fact, it has the potential to help smaller companies and startups punch above their weight. One such example is Quasar Markets, a startup, low-cost data and “lifestyle” platform—meaning that it incorporates all types of content a trader might want access to throughout their day, from weather to sports, and of course financial data, in a single screen—founded by former Money.Net and Benzinga executive Steven Orr.
Built exclusively by using outsourced development organizations, and some third-party tools, the platform will offer a free-of-charge basic option with ads, then a $14.99 per month ad-free subscription, on top of which users can sign up for the datasets they want. But key to the user experience will be personalization enabled by incorporating AI into the platform, Orr says.
The platform will incorporate different types of AI, including predictive AI that can analyze information about a company and other factors that affect it, such as retail trends, to predict price movements and create forward-looking charts, but also a generative AI that can use those predictions to answer users’ questions not only about current market data, but also potential future trends based on abstract ideas, Orr says.
“We may not know the answers, but the AI can make those connections,” he says. “We’re letting the machine do the hard work for us.”
The AI queries official databases, including Fred (Federal Reserve Economic Data), the SEC’s Edgar database, information from the UN and Cloudquant, and more than 50 other datasets ranging from the World Bank to US government departments.
Orr says he anticipates that most users will be asking fairly simple questions of the data, though the platform can also help them become more sophisticated in what they ask by suggesting follow-up questions for the data, which users can simply click to ask, and by also showing what others are asking.
“The AI results are based on real, hard data that tells a story, with links back to the original source data. So you can build your thesis, and by keeping asking questions and asking more questions, you can prove your thesis,” Orr says.
Again, AI isn’t about taking that thesis out of your hands and putting it into the claws of The Terminator. Rather, it’s about freeing up your hands so you can multitask and do more with the information that you’re now receiving faster and clearer than before, and, if you’re using it right and have accurate and correctly labeled data underpinning it, should make you more successful than before.
Of course, you can’t spell “life” without “if”—and those are some big “ifs.” But not ones that can’t be overcome, especially if we embrace AI as an ally.
Thoughts? Case studies? Paranoid ramblings? Send them to max.bowie@infopro-digital.com. Now please excuse me while I go unplug my toaster.
Further reading
Only users who have a paid subscription or are part of a corporate subscription are able to print or copy content.
To access these options, along with all other subscription benefits, please contact info@waterstechnology.com or view our subscription options here: http://subscriptions.waterstechnology.com/subscribe
You are currently unable to print this content. Please contact info@waterstechnology.com to find out more.
You are currently unable to copy this content. Please contact info@waterstechnology.com to find out more.
Copyright Infopro Digital Limited. All rights reserved.
You may share this content using our article tools. Printing this content is for the sole use of the Authorised User (named subscriber), as outlined in our terms and conditions - https://www.infopro-insight.com/terms-conditions/insight-subscriptions/
If you would like to purchase additional rights please email info@waterstechnology.com
Copyright Infopro Digital Limited. All rights reserved.
You may share this content using our article tools. Copying this content is for the sole use of the Authorised User (named subscriber), as outlined in our terms and conditions - https://www.infopro-insight.com/terms-conditions/insight-subscriptions/
If you would like to purchase additional rights please email info@waterstechnology.com
More on Data Management
Waters Wavelength Podcast: The issue with corporate actions
Yogita Mehta from SIX joins to discuss the biggest challenges firms face when dealing with corporate actions.
Data catalog competition heats up as spending cools
Data catalogs represent a big step toward a shopping experience in the style of Amazon.com or iTunes for market data management and procurement. Here, we take a look at the key players in this space, old and new.
This Week: JP Morgan, Broadridge, Lloyds, JSE, Schroders, and more
A summary of the latest financial technology news.
What firms should know ahead of the DSB’s UPI launch
Six jurisdictions have set deadlines for firms to implement the derivatives identifier, with more expected to follow.
Has cloud cracked the multicast ‘holy grail’ for exchanges?
An examination of how exchanges—already migrating to the cloud—are working to solve the problem of multicasting in a new environment.
Waters Wrap: Market data spend and nice-to-have vs. need-to-have decisions
Cost is not the top factor driving the decision to switch data providers. Anthony looks at what’s behind the evolution of spending priorities.
The consolidated tapes are taking shape—but what shape exactly?
With political appetite established on both sides of the Channel, attention is turning to the technical details.
Ignoring ESG data simply doesn’t make business sense
There’s a brewing controversy about “woke” ESG investments. But politics aside, ESG as a dataset brings more transparency to investment decisions.