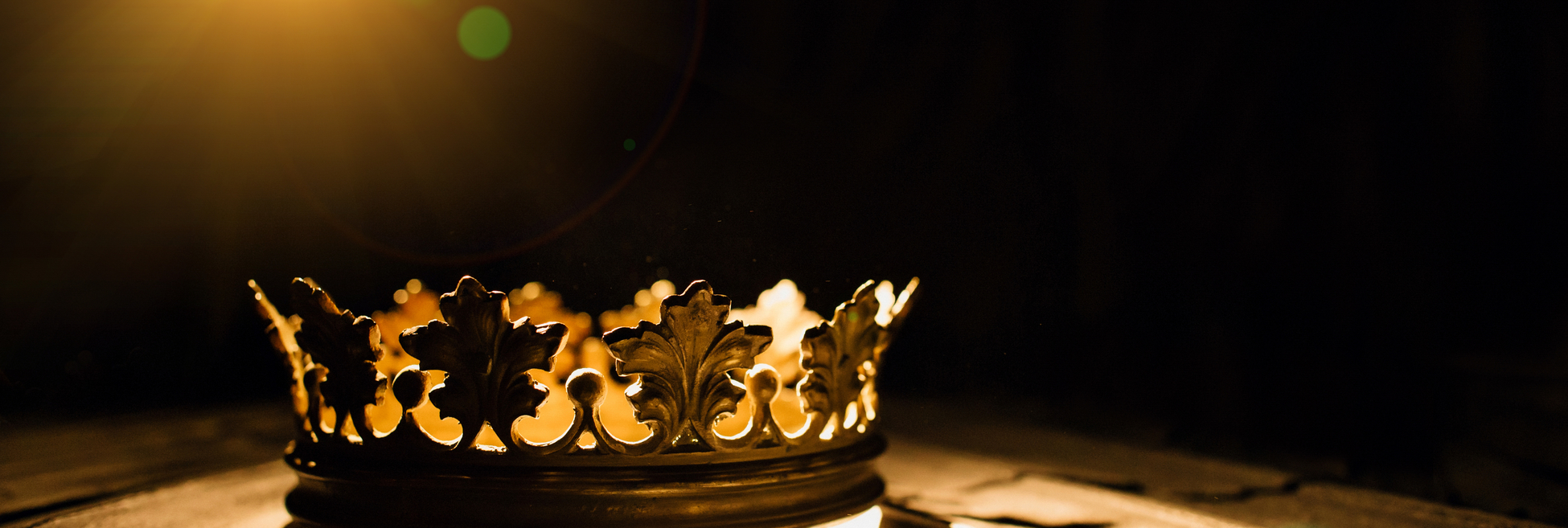
In the world of financial data, context—not content—is the new king
For years, the mantra of the market data world has been ‘content is king.’ But with trading strategies now more dependent on being able to see the big picture, the value of context could quickly overtake the data itself.
They say a picture tells a thousand words. For example, JMW Turner’s 1838 painting The Fighting Temeraire, tugged to her last berth to be broken up, 1838—which depicts the last voyage of the HMS Temeraire, a British warship that fought at the Battle of Trafalgar, to a berth in Rotherhithe to be scrapped—does just that. However, each component of the painting, taken individually, requires the others to complete the scene. Taken alone, each element would be a masterpiece, but would not tell the story of the ship’s last journey. Each requires the context that all the other elements, when taken together, provide—plus the painting’s title.
But at best, every picture, like a single frame of film, is still merely a snapshot of a specific moment in time. If a picture tells a thousand words, the additional context that turns that snapshot into a story tells a thousand more. So to then place that story into a broader historical context and to understand its significance, we need additional contextual information from other sources.
Context isn’t new. It’s something that traders and asset managers have instinctively added from their own experience or sought out to enrich decision-making processes. But whereas in the past it was a nice-to-have, now it’s essential. Because if you want to tell how the market is moving or what’s going to happen next, a data point is basically a “You are here” arrow on a blank page. For it to have value, you need more data, and to be able to connect that data. You need the whole street map.
“Data’s still the fuel in the tank, but context is what gets you where you want to go. Without context, you’re making decisions in a vacuum,” says Bruce Fador, CEO of data and fintech management and sales consultancy firm Fador Global Consulting Group. “It’s always been that way, but it’s becoming more mainstream … especially now in uncertain times. Markets don’t like uncertainty, so asset managers are saying, ‘What do I do?’”
Don’t just give me data. Tell me a story
It’s a sentiment that resonates with Saad Hussain, founder and CEO of California-based Vesica Technologies, which provides a tool called Shift Search that displays open-interest and volume data, broken down by put and call activity, along with relevant news. Last year, the Miami International Securities Exchange (Miax) adopted Shift Search as a central component of its Virtual Trading Floor information portal. Hussain realized there was more value beyond the data itself, and that by combining datasets, he could provide valuable contextual information.
Before founding Vesica, Hussain worked as an oil options broker for almost a decade at PVM Oil Associates (now owned by TP Icap), and clients would constantly call him, asking for his summary of the market. That was part of a broker’s role—to weave together all relevant information and context to give clients everything they needed to make a decision. The clients could have looked the data up themselves, but they wanted more insight than they could achieve alone. That’s when he realized that “people want context, not just a data point. You have to tell people a story. The challenge is, how do you allow people to see that story without being too complex about it?”
For example, headline put and call information provides a snapshot in time of options activity, but Shift Search also allows users to look at historical put and call activity. “So, for example, if you see a bunch of call option buying, you can see that ‘story’ being formed,” Hussain says. “How open-interest, volatility, and money flows behave all tell a story.”
Fador’s previous employer, corporate earnings data analytics provider Wall Street Horizon (WSH), where he served as chief commercial officer between 2014 and 2017 and remains an adviser, understands the value of using data to tell a story, rather than just presenting raw data points.
“Context helps develop a narrative about a company. So for buy-side investors, it’s all about context,” says WSH CEO Barry Star. For example, understanding why a company does something, and what that something means, is potentially more valuable than simply knowing that a company has done something in the first place.
“Data alone is less valuable than data in context,” he says. “There’s a big difference between data, information, and knowledge. Data is just that—bits and bytes: trade data, tick data, or earnings data—an event. If you take data and add value—if you transform it—it becomes information. So, if an earnings date is data, then when an earnings event changes its date, that’s information. Putting data in context adds value. So, if you take information and add value, it becomes knowledge.”
WSH’s business is all about providing context: not just confirming the date on which a company will announce results, but whether that date is earlier or later than usual, and whether that indicates good or bad news. For example, a date is a date. But if a company schedules a corporate event such as an earnings announcement in a way that’s completely different from its past behavior, and if an observer has the historical information to understand the historical significance of that schedule or date change, “that’s context—that’s knowledge,” Star says.
Other examples could include if electric car manufacturer Tesla starts running a new ad campaign, or if the CEO of a company buys 10,000 shares in company stock. That context is knowing that, for example, Tesla famously doesn’t spend money on advertisements or endorsements, or that the company CEO has never previously bought shares on the open market.
WSH’s data is a well-known example of alternative data that adds context. But context comes in all shapes and sizes, and can be derived from many types of sources.
Aside from the much-hyped and highly valuable alternative data industry—typically information from other industries that can serve as an additional input to trading decisions in financial markets—one source of context is sentiment data, assessing investor (both retail and institutional) sentiment on a company or stock based on analysis of how news, research, blogs, social media posts and other market activity historically impacts share prices. Sentiment is pretty well established, but now some are taking it a step further.
One of these is Buck Andrews, an industry veteran who has been a hedge fund manager, founded Omniquant, and served as president of data company AT Financial, which was sold to Thomson Corp in 1997. Now, he has co-founded a new company, In4m (pronounced “Inform”), developed with a former executive of now-defunct hedge fund Pequot Capital Management and an artificial intelligence (AI) expert at the University of Sydney, which uses AI to categorize and add context to news articles.
“Everyone has content. There’s so much that it’s hard to process. We want to contextualize information and make it easy to consume,” Andrews says.
In its most basic form, In4m’s product uses AI to categorize news items—serving as a productivity tool that could save research analysts hours each day over manually digging up news items relating to a portfolio company—and assess the impact that each item could have on stock prices. A second iteration of the product, expected in Q3 this year, will incorporate third-party macroeconomic and sector research to provide more context around the impact of news items on prices.
“We’re providing the news items, but also layering in context to the data that will help people—probably not high-end hedge funds, even though it partly came out of a hedge fund, but potentially anyone investing in individual stocks or individual sectors—invest in the right sectors and recognize the economic cycle they’re in,” Andrews says.
Oldies but goodies
But those providing new ways of adding context aren’t necessarily just new startups or alternative data vendors. In fact, in some cases—depending on the type of data—established, traditional data vendors and exchanges are best placed to add context, because they have access to the underlying market data and fundamentals to which context adds value.
For example, another company making the most of opportunities to add value to news is MT Newswires, founded in 1999 by CEO Brooks McFeely.
“Context is probably one of our most significant differentiators. Our mission is to provide not just pricing coverage, but context and perspective,” McFeely says. “For example, we provide coverage of earnings, but we also provide information on past earnings, and historical coverage of how the market prices earnings news into a company’s stock price. So, we look at context as an essential part of what we do. We have to tell the market what’s happening, when it’s happening, and why it’s happening … and provide a historical perspective so the market can make well-informed decisions.”
But, McFeely adds, providing the most valuable context isn’t always about adding extra information. Sometimes the best way to provide context is to eliminate information that’s just noise to give a clearer view of the information that’s genuinely useful.
“We’re not deciding what information investors should know or not know; we’re just filtering it for relevance,” he says. For example, insider trading activity is something investors deem useful, but is hard to correlate against price action. “Is it really interesting to know that someone has sold $5,000 of Google stock for tax purposes?” he says. So instead of just reporting on insider trades, MT Newswires also reports on aggregate transactions in a company and across industry sectors, to identify trends and show how one company’s insider activity compares to that of its peers.
Arizona-based data terminal and datafeed vendor QuoteMedia takes a similar approach to insider transactions, not just flagging when a company executive buys or sells stock, but also showing how their holdings have changed over time, and trying to ascertain why insiders might be, say, buying a lot of stock in their company at a certain time. The vendor also compares this activity to other companies in a user’s portfolio to determine whether this is unusual activity, so the user can decide whether they want to adjust their holding in that stock.
But for QuoteMedia, one of the keys to adding value through context is by providing “data on the data,” and highlighting where the most value exists, says CEO Dave Shworan. Based on its historical data, the vendor computes confidence scores that predict how profitable a user’s chosen options trading strategy will be, or to highlight stocks that have potential for movement. The vendor computes “running scores” in real time, and also provides up to 10 years of historical scores so users can analyze trends in the scores, sub-scores, and underlying data metrics. These include hundreds of individual metrics—items such as capital efficiency, debt vs assets, net debt, and return on equity.
“So, if someone is looking at their portfolio or researching stocks, they can get computer-generated information that tells them what they should be looking at,” Shworan says.
Go with the flows
A recent report from consultancy Aite-Novarica Group, titled New edges: How to make the most of trading flow data, highlights some examples of what types of contextual data can add value, where firms can find it internally, and the challenges of gaining access to it.
For example, the report cites how a firm’s municipal bonds trading desk could benefit from information from a retail mortgage lending division: an increase in mortgages for new home purchases in specific zip codes would result in higher tax revenues for the local municipality, which might result in a higher credit rating. Conversely, being aware early of defaults on mortgage payments by multiple borrowers in a zip code could be used as a risk alert to adjust the firm’s strategies.
Aite-Novarica’s report identifies similar synergies between fixed income and equities trading desks, corporate actions processing functions and equities desks, and client onboarding across different parts of an organization. In short, a firm’s own existing data flows have the potential to enrich trading activities in other parts of the same firm and generate alpha. In addition, because the data is internally derived, it doesn’t result in increased data license fees.
However, that doesn’t mean it doesn’t come with a cost. The research notes that getting access to the right data—even data that already exists and is available within their own firm—can pose significant challenges. Sometimes these challenges are technical and revolve around the internal infrastructure and data platforms required to extract data from one business line and make it available to another in a manner timely enough to take full advantage of the data, with all of the associated controls and permissioning.
“A division may be trying to get access to what they don’t have today, to open up possibilities around what they can do with the data. But opening up those opportunities requires investment, and for that, you need to make a business case,” says Vinod Jain, senior analyst for capital markets at Aite-Novarica, who authored the report. “But the ‘data owner’ doesn’t want to create an expensive, long-term project—they just want the data. So, they want solutions that integrate with their existing technology platforms.”
To ease the technical challenges, many firms have built—or are building—central risk books (CRBs) that allow different parts of a firm to see exposures and activity in other areas, says Ishaan Nerurkar, CEO of LeapYear Technologies, whose platform enables secure and compliant data sharing across organizations. But the challenges can also be compliance-related. Using the raw data generated by one part of a large bank in other areas of the same firm or externally can create conflicts of interest and risks related to sharing individual customer data.
“There are concerns on the buy side that banks’ CRBs are essentially enabling prop trading. To mitigate these concerns, many market makers have created information barriers, so that, for example, the CRB can’t see agency flows, but can only see what’s trading on its internal desks,” Nerurkar says. “Our technology ensures that all the quants building models inside the CRB can’t learn anything about an individual client’s activity, but can still use aggregated data to make predictions at the individual security level. So, for example, they could see how overall trading activity is expected to change over the coming days, but they can’t see that the bank has a specific client trying to place a specific trade.”
Once that privacy layer is in place, it opens the door to other potentially profitable ways that banks seeking to monetize proprietary data can add context for buy-side clients without violating their obligations.
“Many large banks have big retail businesses as well as their capital markets operations. And the retail side has, for example, credit card data and mortgage pre-payment data, while the institutional side has payments information. Together, that’s a lot of information that would be useful to the buy side for understanding sales of product categories, macro trends, or even as competitive information for merchants themselves,” Nerurkar says. “So, we’ve been working on use cases to surface information from outside of the trading organization and make it available to clients—for example, being able to provide company data to the buy side in a way that protects cardholders and merchants by not disclosing personally identifiable information or information about a merchant who is banking with the firm.”
LeapYear’s examples center around bank-owned datasets. But banks aren’t the only ones looking for ways to extract more value from—and add context to—data within their organizations. In some cases, that necessitates owning the data in the first place to be able to use it for the purpose you want.
Having access to the data that’s needed was one of the drivers behind the decision of low-latency feed handler and ticker plant appliance vendor Exegy to buy Vela, which provided aggregated datafeeds and a market data platform, among other things. The vendor has since integrated its product lines and uses the Exegy appliances to collect low-latency data at source to create the Vela consolidated feeds. These then become the low-latency data input for Exegy’s Signum product suite—a service that generates predictive signals of price movements, and which can trigger automated execution of trading strategies.
Exegy CTO David Taylor says the additional context that services like Signum provides is one of the most exciting areas of development at the moment because of the potential to unlock hidden information about hidden institutional orders and drive trading strategies, but warns that some firms need some initial hand-holding to get the most out of the data.
“There was a segment of our clients who immediately ‘got’ it and had already identified use cases of how they would use it—and they comprised our early adopter sales—then there was a segment who ‘got’ it but hadn’t identified how they would use it on day one, and who asked us to come back with examples of how to use Signum for their business,” Taylor says. So, the vendor hired an expert to help convert the predictive signals into execution algorithms—essentially taking over the heavy lifting associated with understanding the meaning of context from clients.
“The hard part about market data is that it’s mission-critical and relentless in nature. Most clients don’t want to have to worry about that; they just want to be able to plug it in and have it work,” Taylor says. “Mastering the data science, understanding market structure, and creating context that adds value is much harder than just writing feed handlers.”
But not only does this potentially make Exegy’s combined offering more valuable and “sticky” to end-user clients, it also differentiates the vendor from its traditional low-latency technology rivals because the company can deliver both context and price data synchronously, he adds.
Tools of the trade
Differentiation is also a key motivator for exchanges to wade into this space, especially since they are perhaps in the best position to provide context around market data, because they are generally the original source of where market data is generated—at least, in exchange-traded markets—and thus the point where context could be added earliest in the data lifecycle, potentially undercutting those seeking to add value later in the data’s journey.
For Cboe Global Markets, recently integrated acquisitions such as Hanweck and Trade Alert are helping the exchange to add valuable context around its prices, such as how markets have historically priced in volatility in a stock or option based on the issuer’s corporate events.
“Aside from providing prices, we also calculate implied volatility. We put that in context by not just providing what’s implied from an earnings announcement, but we also provide how it has historically behaved—is the market pricing in more or less risk?” says Kevin Nichols, head of derived data and analytics at Cboe. “Also interesting is the order flow into options around an event—are they in or out of the money? Trade Alert does a good job of providing that.”
Another way to add context to the total exchange-traded volumes is to break them down by investor type, so users can see how different segments of the market are behaving. Cboe has an open/close activity dataset that provides this, and has been upgraded in recent years to include order flow data that wasn’t previously available. The data is available as a file download, but Nichols hopes to make it available via other Cboe platforms and via an API, subject to client demand.
Why is this data useful? “With the growth of the retail trader, you have to pay attention to that segment. I’ve never seen anything like the GameStop incident. They’re a decent force in the marketplace now,” Nichols says. Clients can also use the dataset to watch for increases in open interest that signify someone taking larger positions, or for increased activity around market close, which would indicate traders winding down positions.
“My role is focused on bringing out data from our exchanges that doesn’t currently exist—and there’s still a lot of opportunity to do that,” he says.
Canadian exchange group TMX also sees plenty of opportunity to add value at the exchange level.
“We have our core content—data—and we’re trying to move up the value chain to knowledge and insight, which gives our clients the ability to generate alpha and manage risk,” says Michelle Tran, president of TMX Datalinx, the exchange’s data business.
For example, TMX has recently soft-launched new data products, including investor flows data, which uses AI and machine learning to provide insights into aggregate retail trading activity and equity market momentum, and liquidity ratings, which provide security-level indicators of the cost to trade based on bid–ask spreads, market depth, and quote resiliency. In addition, TMX plans an “Enriched FIPS” fixed income pricing service incorporating analytics such as yield-to-maturity, duration, relative-value spread, and evaluated price.
“We believe it is important to provide the financial data and the associated metadata (which is the information about the data) so that our clients can better connect these datasets and derive new insights to inform their investment and trading processes,” Tran says.
The ability to access and connect disparate datasets to generate knowledge and insight is one of the drivers behind the exchange’s increased adoption of cloud computing in its data operations. Yes, the cloud offers a modern way to acquire and onboard clients and allow them to connect to exchange data without expensive legacy infrastructure technologies. But it also represents an important tool that makes it easier for the exchange and others to link unrelated datasets.
“We view the cloud as helping to enable seamless access to data for our clients and partners. We are launching our core pricing, index, and reference data into the cloud, as well as the contextual data such as investor flows, liquidity ratings, enhanced fixed income and others so that our clients can easily access this data and connect these datasets to enable new sources of investment edge,” Tran says.
“Delivering our market data to established cloud service providers (CSPs) provides TMX with the opportunity to approach and acquire new clients that we’re otherwise unable to directly reach today—digital-native companies that operate with an entirely agile and cloud-first go-to-market strategy. Importantly, the public cloud also allows clients to integrate TMX content with toolsets that can conflate, overlay, and analyze TMX data with a multitude of third-party content sets.”
Cloud may indeed prove key to context because cloud is also an important enabler of the AI and ML tools used by TMX, and other technologies. Ultimately, all these contribute to developing new sources of contextual data that provide better insights for all data consumers.
“Relevant information comes from having context. And to get that context, you need tools,” such as AI that enriches raw data with context in real time to provide immediate access to useful insights that a professional would have otherwise needed to piece together themselves and gather information from their own sources, Fador says. “Tools are helping people be smarter about the data they’re looking at, and context broadens the capabilities of all of us.”
Ultimately, those adapting their businesses from providing raw data to providing value-added context around that data are making the smart move toward preserving and even growing data revenues as traditional, basic price data becomes commoditized.
As Vesica’s Hussain says: “I believe the value of data will go down, but the value of context will go higher.”
Only users who have a paid subscription or are part of a corporate subscription are able to print or copy content.
To access these options, along with all other subscription benefits, please contact info@waterstechnology.com or view our subscription options here: http://subscriptions.waterstechnology.com/subscribe
You are currently unable to print this content. Please contact info@waterstechnology.com to find out more.
You are currently unable to copy this content. Please contact info@waterstechnology.com to find out more.
Copyright Infopro Digital Limited. All rights reserved.
You may share this content using our article tools. Printing this content is for the sole use of the Authorised User (named subscriber), as outlined in our terms and conditions - https://www.infopro-insight.com/terms-conditions/insight-subscriptions/
If you would like to purchase additional rights please email info@waterstechnology.com
Copyright Infopro Digital Limited. All rights reserved.
You may share this content using our article tools. Copying this content is for the sole use of the Authorised User (named subscriber), as outlined in our terms and conditions - https://www.infopro-insight.com/terms-conditions/insight-subscriptions/
If you would like to purchase additional rights please email info@waterstechnology.com
More on Data Management
The IMD Wrap: Talkin’ ’bout my generation
As a Gen-Xer, Max tells GenAI to get off his lawn—after it's mowed it, watered it and trimmed the shrubs so he can sit back and enjoy it.
Waters Wavelength Podcast: The issue with corporate actions
Yogita Mehta from SIX joins to discuss the biggest challenges firms face when dealing with corporate actions.
Data catalog competition heats up as spending cools
Data catalogs represent a big step toward a shopping experience in the style of Amazon.com or iTunes for market data management and procurement. Here, we take a look at the key players in this space, old and new.
This Week: JP Morgan, Broadridge, Lloyds, JSE, Schroders, and more
A summary of the latest financial technology news.
What firms should know ahead of the DSB’s UPI launch
Six jurisdictions have set deadlines for firms to implement the derivatives identifier, with more expected to follow.
Has cloud cracked the multicast ‘holy grail’ for exchanges?
An examination of how exchanges—already migrating to the cloud—are working to solve the problem of multicasting in a new environment.
Waters Wrap: Market data spend and nice-to-have vs. need-to-have decisions
Cost is not the top factor driving the decision to switch data providers. Anthony looks at what’s behind the evolution of spending priorities.
The consolidated tapes are taking shape—but what shape exactly?
With political appetite established on both sides of the Channel, attention is turning to the technical details.