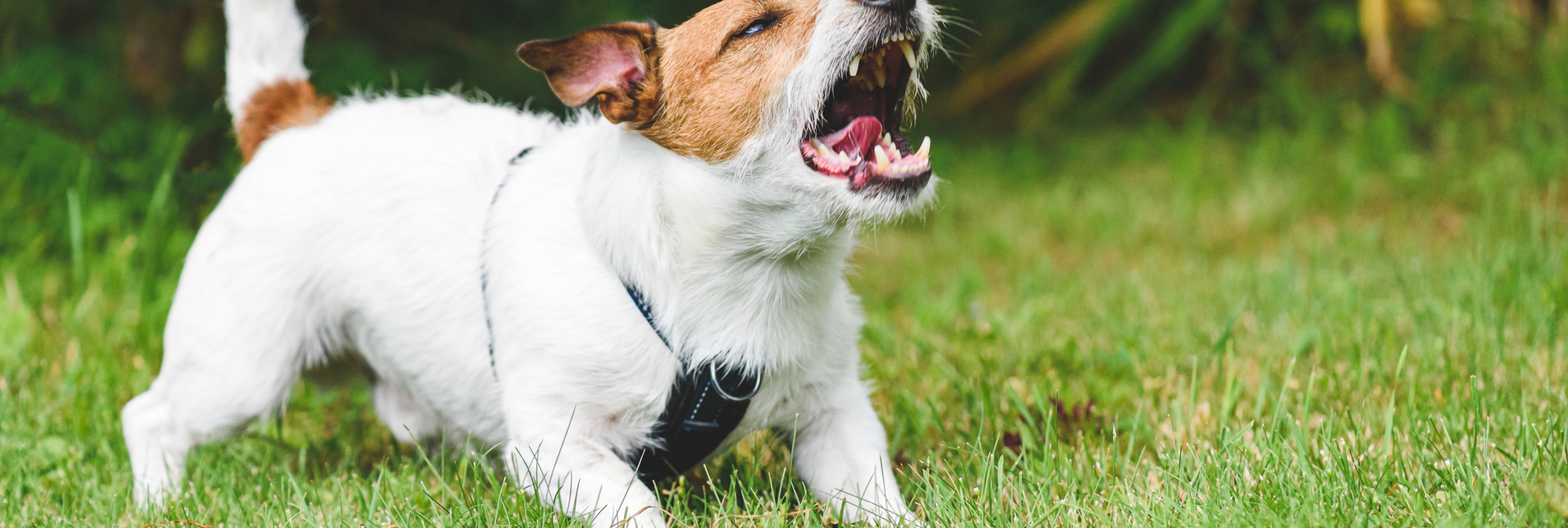
Banks’ internal watchdogs bark back at ChatGPT
Generative AI has plenty of uses in finance, but banks must first overcome compliance headaches
Need to know
- Banks are keen to test the capabilities of generative AI such as ChatGPT for producing content ranging from coding to client briefings.
- But they may not be early adopters because of severe operational risks that could result in regulatory enforcement or customer litigation.
- The possibility of generative AI producing false outputs dubbed ‘hallucinations’ is alarming, and all outputs will need to be explained to senior managers and supervisors.
- The use of third-party AI model providers will also raise questions about data security and intellectual property rights.
Little has sparked more media excitement than ChatGPT over the past few months. Powered by an underlying technology known as generative artificial intelligence (AI), based around predicting complex patterns, the chatbot has charmed and unnerved in equal measure with its ability to engage in almost-human conversations and perform non-linear tasks, from writing research papers to producing software code.
The financial sector, including Wall Street banks, has also recognized the significance of this moment, with many of the largest banks appointing senior executives to lead AI initiatives over the past 12 months.
“There is interest right from the top of the house across the board, and we are keen to push this technology through,” says the head of AI at one global bank.
Several large banks are now engaged in exploratory projects featuring generative AI, often partnering with the biggest technology providers. Morgan Stanley is working on a project with OpenAI, the inventor of ChatGPT itself, while Deutsche Bank is exploring the possibilities of Google’s generative AI tools.
But large financial institutions are rarely early adopters of new tech and they are unlikely to be at the forefront of building applications for generative AI. That’s because they are also some of the most highly regulated institutions, leaving in-house compliance and legal teams with a lengthy list of potential risks to its adoption that must be overcome. And given the challenges to using the technology in a compliant way, simply entertaining the kids with AI-generated text isn’t good enough, either—banks also want to see a rigorous analysis of use cases.
While that evaluation exercise is ongoing, an informal survey by WatersTechnology’s sibling publication Risk.net has found that at least six global systemically important banks (G-Sibs)—JP Morgan, Bank of America, Citi, Goldman Sachs, Wells Fargo and Deutsche Bank—have temporarily curbed the use of ChatGPT among employees. Morgan Stanley allows limited personnel access, only for pre-approved use cases under rigorous governance and controls.
“We are still at an exploratory stage to ensure the technology aligns with our risk appetite and regulatory requirements. We want to use it in a way that we can explain the technology internally to ourselves and create real economic values for our clients,” says the head of AI at the global bank.
Among 16 banks, AI experts and consultants spoken to for this article, all unanimously identified four risks as top priorities: data security; copyright issues; explainability; and ‘hallucination’.
“Addressing the risks to make generative AI compliant and usable should be an extremely high imperative for banks, and those who can figure it out first are going to have a competitive advantage moving forward,” says a senior quant at a second global bank.
Do androids dream of earnings reports?
Perhaps the most alarming potential mishap of generative AI is its ability to simply make things up, often referred to as ‘hallucination’. Just as we humans can experience sensory illusions, AI models hallucinate when generating outputs that appear plausible but are actually inaccurate or unrelated to the given input context.
A risk management analyst says she used ChatGPT to write a policy analysis report, but found it generated fabricated citations and references. Similarly, a financial analyst tried the chatbot to draft earnings reports. It reads well, he says, but some of the information was fundamentally flawed.
While tech companies are racing to improve the accuracy of their models, hallucination is still listed as a limitation on the product page for OpenAI’s latest AI model, GPT-4, as well as on Google’s Bard website.
“There is no silver bullet for addressing the risk because it is a fundamental issue with the way generative AI is built,” says Raj Bakhru, chief strategy officer at ACA Group.
Unlike traditional AI models that rely on predefined rules or specific input-output mappings, generative models such as ChatGPT learn from an extensive corpus of datasets on the internet and generate novel content. More specifically, they are trained to do one thing in particular: predict the next word in the sentence.
For instance, when given a prompt such as “the risk of generative AI is X”, ChatGPT will use a probability distribution to calculate potential words, and selects one based on its calculation. The distribution may assign higher probabilities to words like ‘high’, ‘significant’ or ‘unpredictable’, as they are commonly associated with the given prompt. That said, the model does not always select the word with the highest probability—its decision is based on how it is trained by the developers. This unsupervised learning pattern, as a result, leads to unpredictable outputs.
“The model is not trained for a confidence interval, but for optimizing response. So it has no sense of whether the response is truthful or not,” says Christine Livingston, managing director of innovation and AI at consultancy Protiviti.
A senior model risk expert at a third global bank suggests a phased approach to mitigate the risk. First, start with use cases targeting ‘internal experts’, including existing risk managers and model developers, who have a clear understanding of their requests to the chatbot. By leveraging the expertise of these individuals, banks can gather valuable feedback and validate outputs to better refine the model.
Once the model demonstrates a sufficient level of maturity, the model risk expert says, banks can gradually expand the usage to include internal non-experts, enabling the model to handle a wider range of queries and scenarios, and benefit more employees.
Addressing the risks to make generative AI compliant and usable should be an extremely high imperative for banks, and those who can figure it out first are going to have a competitive advantage moving forward
Senior quant at a global bank
For instance, Morgan Stanley is actively testing its internal GPT with over 800 financial advisers and support staff before deploying it to the entire wealth management team.
Eventually, as the model continues to evolve, it can be extended to external clients in certain specific use cases, such as providing investment strategies.
The senior quant at the second global bank says the model will require not only ongoing testing and training to enhance its accuracy, but also proper guidance to bank employees on developing effective prompts.
“The more specific context you give in the prompt, the more likely you will get accurate answers,” says the quant.
Furthermore, as with conventional model risk management, he thinks firms should deploy challenger models to evaluate the generative AI’s responses.
“You can ask the generative model to give you multiple answers and then get a different model to evaluate which one is the best result,” he says.
How to tell the boss
Closely allied to the risk of hallucination is the need to be able to explain model functioning and outputs to all stakeholders, including board members and supervisors.
While explainability has long been a hurdle to AI models’ adoption among banks, it becomes particularly pronounced when dealing with generative models with complex architecture and huge numbers of inputs.
“GPT-3 is a 175 billion parameter model—how much can you actually explain? Not to mention it will only get bigger and bigger,” says the head of AI at the first global bank.
These problems would be even more severe where banks are using third-party models.
“How are banks going to explain the model when they don’t even have access to its underlying algorithm and code?” says Brian Consolvo, principle of technology risk management at KPMG.
The models’ inner workings and learning patterns add further complexity. Most generative models apply ‘embedding’, a technique used in machine learning and natural language processing. This converts input data, such as words, sentences or images, which neural networks cannot directly process, into numerical representations. These embeddings capture the semantic meaning and contextual information, allowing the model to generate coherent output.
However, unlike tabular data where the input variables have clear interpretations—such as income, age and education level in a typical credit risk model—these numerical factors do not have specific meanings, making it exceedingly challenging to explain, says the model risk expert at the third global bank.
Nonetheless, he believes there are additional statistical techniques that banks can employ to enhance explainability when they lack model visibility. Although existing methods are not yet directly applicable to generative models, he believes that progress will be made soon, specifically by monitoring which parameters of the model are activated for generating each output.
“The industry is working furiously on this,” says the model risk expert. “Within a year or two, you will see a lot of development in this area, and people will become more confident in explaining generative models.”
In the meantime, the head of AI at the first global bank suggests firms will need to be very selective on the use cases for generative AI. For example, explainability is less of a concern if the AI is deployed to help write code.
“Before code goes into production, it must undergo a solution delivery lifecycle, which includes unit testing, integration testing and user acceptance testing,” says the head of AI. “These clear procedures make it easier to quantify the output, giving a level of transparency and control for us to incorporate the coding-related outcomes of generative AI into our workflow.”
The model is not trained for a confidence interval, but for optimising response. So it has no sense of whether the response is truthful or not
Christine Livingston, Protiviti
Several sources suggest knowledge management is another activity that has a low explainability risk. In this activity, generative AI tools are primarily used for relatively constrained functions, such as helping to locate and summarise content based on rigorous controlled input data, rather than making complex decisions.
By contrast, using AI for decisions such as retail credit underwriting is a much higher-risk activity from a legal and reputational perspective.
“Especially in the consumer space, you can’t even go down this path if you have challenges with explainability,” says Sameer Gupta, advanced analytics leader for financial services at consultancy EY.
Even for investor clients, supervisory scrutiny could become intense. In its spring regulatory agenda, the US Securities and Exchange Commission indicated that it plans to propose rules “related to broker-dealer conflicts in the use of predictive data analytics, artificial intelligence, machine learning and similar technologies in connection with certain investor interactions”.
Mind your data
Another aspect currently facing a tougher stance from regulators is data security, with the SEC proposing a new set of rules on cyber security in March this year, and third-party technology companies under the microscope after the cyber attack on trading software provider Ion at the end of January. This poses particular compliance questions for ChatGPT and other hosted generative AI packages.
“Like other banks, we have currently blocked the [ChatGPT] website for all staff as we evaluate how to best use these types of capabilities while also ensuring the security of our clients’ data,” says a spokesperson at Deutsche Bank. “It is about protection against data leakage, rather than a view on how useful the tool is.”
Corporate users are particularly worried about how third-party chatbots handle their data. In March, right after Samsung’s semiconductor division allowed the use of ChatGPT, its employees were reported to have shared confidential information with the chatbot on multiple occasions, raising concerns that it could be used by OpenAI for future model training.
“This is a big risk. In an extreme case, you could find yourself in violation of a non-disclosure agreement. For banks, you may run afoul of regulators,” says Jeremy Tilsner, senior director at Alvarez & Marsal’s disputes and investigation unit.
In wake of user concerns, OpenAI has tightened up ChatGPT’s data management policy. Since April, users have the option to disable chat history to ensure their input is not used for model training. Additionally, the AI firm plans to roll out a new ChatGPT business subscription in the coming months, specifically tailored for professionals who require greater control over their information and data. Under the subscription, input data would not be used for training purposes by default.
Despite OpenAI’s effort, sources say the risk remains elevated if any confidential information leaves corporate environments, particularly for banks, which house vast amounts of proprietary and sensitive data. Any leaks of client data could expose the bank to significant legal risks.
“Firms like Microsoft claim they will keep users’ information confidential. But what happens if a rogue employee exposes sensitive client information, or a bug in the system is exploited by hackers?” asks the senior quant at the second global bank.
In March, OpenAI confirmed a bug in ChatGPT’s source code that allowed some users to view other users’ chat history. Further investigation suggested the same bug may have leaked payment-related information for more than 1% of ChatGPT Plus subscribers who used the chatbot during a specific time period. While OpenAI patched the bug, and claimed to have taken additional steps to improve the system, the incident underscored public apprehension surrounding third-party security risk.
“Banks need to prioritize evaluating data security risk before delivering into specific use cases,” says EY’s Gupta.
One obvious solution is to keep the data within the bank’s own security environment. This can be achieved through collaboration with vendors to develop internal chatbots, as seen with Morgan Stanley’s partnership with OpenAI, or by taking the safest approach of building in-house generative AI systems.
How are banks going to explain the model when they don’t even have access to its underlying algorithm and code?
Brian Consolvo, KPMG
“Banks will increase the accuracy and reduce the risks by hosting their own generative AI, but that takes time, effort, resources, money and skills that they may not have today,” says Michael Silverman, vice-president of strategy at the Financial Services Information Sharing and Analysis Center.
For this reason, the head of AI at the global bank expects a mix of in-house and third-party AI. In addition, nearly all banks interviewed for this story are leaning towards building smaller generative models tailored for specific needs, rather than large language models (LLMs) such as ChatGPT.
“I would say there are no more than 10 banks in the US who have the capacity to invest and build their own LLMs,” says Gupta. “And even for them, is it really the best thing to do?”
Indeed, there is a growing recognition among banks that larger models may not necessarily yield better results from an efficiency standpoint.
For instance, in cases where banks solely require generative models for information retrieval, the ability of the LLM to generate lengthy and intricate sentences or produce novel content becomes unnecessary.
“A lot of [the] time, 13 billion to 65 billion parameter models are good enough. We don’t really need an excessively large model like ChatGPT,” says the model risk expert at the third global bank.
Whose data is it anyway?
Outsourcing elements of generative AI models raises a further risk: uncertainty around intellectual property rights. Reporting for this story shows that several banks initially used ChatGPT to adjust, rewrite or convert code, but gave it up over copyright concerns.
“Converting code from R to Python manually is a painful task, so that’s why many people at banks are pushing for the use of ChatGPT. But the big question here is: who owns the new code?” asks a senior operational risk manager at a fourth global bank.
He notes that when companies use a translator for a human language—for instance, translating English into Japanese—there is no impact on the copyright. But converting code is not so straightforward.
“It sometimes goes beyond translation, and involves code adjustment and creation to ensure functionality, so I could see some lawyers trying to argue on this and claim the output generated by tools like ChatGPT is not owned by banks,” he says.
According to ChatGPT’s current policy, OpenAI assigns to users “all its right, title and interest in and to output”, which means that users can put it to any purpose that applicable law permits. But bank legal teams worry the policy is subject to change in the future as OpenAI’s own business model evolves.
The operational risk manager says OpenAI could reassure banks by agreeing clearly worded contracts. Until then, banks are “afraid of using third-party tools like ChatGPT to convert code”, he says.
Even if a bank is building an in-house model, copyright concerns persist. Specifically, firms will need to be aware of the sources of their input training data as they broaden the use cases for generative AI.
“When the training data holds value, data providers such as Reddit and Stack Overflow may start to think: ‘Hang on a bit, my data is worth money.’ So they will demand copyright protection on their sources,” says the senior quant at the second global bank. “It wouldn’t surprise me if there are challenges to auditing model inputs in the future.”
The head of AI at the first global bank holds a more optimistic view on this matter, saying that his team keeps copyright issues in mind while testing the technology: “Some of these things will be ironed out as the technology matures.”
Despite the compliance challenges, that optimism is widely shared, with the model risk expert saying the “dust will settle” as the industry develops a better understanding of the necessary controls and the optimum uses of generative AI.
“Banks are always cautious in adopting new technology,” says the senior operational risk manager. “It took a decade for them to fully trust cloud computing, and they are restricting ChatGPT and relevant technologies for now, but, ultimately, they will embrace them, given the effectiveness of those tools and the competitive pressure out there.”
Only users who have a paid subscription or are part of a corporate subscription are able to print or copy content.
To access these options, along with all other subscription benefits, please contact info@waterstechnology.com or view our subscription options here: http://subscriptions.waterstechnology.com/subscribe
You are currently unable to print this content. Please contact info@waterstechnology.com to find out more.
You are currently unable to copy this content. Please contact info@waterstechnology.com to find out more.
Copyright Infopro Digital Limited. All rights reserved.
You may share this content using our article tools. Printing this content is for the sole use of the Authorised User (named subscriber), as outlined in our terms and conditions - https://www.infopro-insight.com/terms-conditions/insight-subscriptions/
If you would like to purchase additional rights please email info@waterstechnology.com
Copyright Infopro Digital Limited. All rights reserved.
You may share this content using our article tools. Copying this content is for the sole use of the Authorised User (named subscriber), as outlined in our terms and conditions - https://www.infopro-insight.com/terms-conditions/insight-subscriptions/
If you would like to purchase additional rights please email info@waterstechnology.com
More on Emerging Technologies
Quants look to language models to predict market impact
Oxford-Man Institute says LLM-type engine that ‘reads’ order-book messages could help improve execution
The IMD Wrap: Talkin’ ’bout my generation
As a Gen-Xer, Max tells GenAI to get off his lawn—after it's mowed it, watered it and trimmed the shrubs so he can sit back and enjoy it.
This Week: Delta Capita/SSimple, BNY Mellon, DTCC, Broadridge, and more
A summary of the latest financial technology news.
Waters Wavelength Podcast: The issue with corporate actions
Yogita Mehta from SIX joins to discuss the biggest challenges firms face when dealing with corporate actions.
JP Morgan pulls plug on deep learning model for FX algos
The bank has turned to less complex models that are easier to explain to clients.
LSEG-Microsoft products on track for 2024 release
The exchange’s to-do list includes embedding its data, analytics, and workflows in the Microsoft Teams and productivity suite.
Data catalog competition heats up as spending cools
Data catalogs represent a big step toward a shopping experience in the style of Amazon.com or iTunes for market data management and procurement. Here, we take a look at the key players in this space, old and new.
Harnessing generative AI to address security settlement challenges
A new paper from IBM researchers explores settlement challenges and looks at how generative AI can, among other things, identify the underlying cause of an issue and rectify the errors.