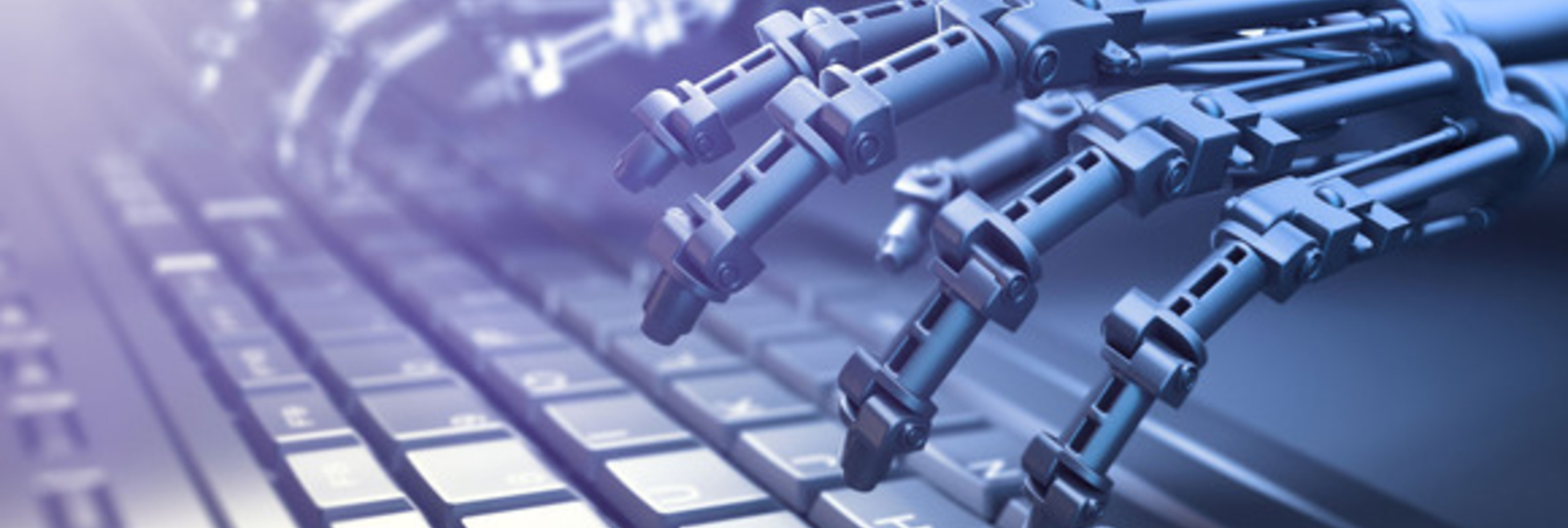
Automation in fixed income: Sorting the bluster from the bleeding edge
Automation is gaining traction in fixed-income markets, but use cases are limited and progress is slow. Market participants must overcome some uniquely human problems before they fully embrace the machine.
The word “automation” has been rattling around financial markets for decades, and the rapid development of AI and machine learning (ML) has only increased the chatter of late. Even in the world of fixed income, where modernization has traditionally been slow to arrive, excitement about the potential of automation is positively boiling over.
And no wonder—the scale of the opportunity is vast. With millions of active securities from dramatically different asset classes scattered across increasingly fragmented liquidity pools, the fixed-income market is a giant data puzzle crying out to be solved. Those who crack it first could reap big rewards in the form of more accurate pricing, enhanced liquidity discovery, better execution, and reduced reliance on specialist programmers.
But the rate of adoption is not as fast as anticipated. Take AI, for example. The term has been bandied around at fixed-income conferences for more than a decade. Still, a recent study by Broadridge found that executives at major financial institutions, if given a magic wand and tasked with accelerating transformation, would first “dramatically expand the use of AI across the enterprise.”
In places where advanced technologies are used, their prominence is often exaggerated. Audrey Blater, a senior analyst at research firm Coalition Greenwich, put together a report on the use of AI and ML in fixed income. While such technology has interesting potential applications, she explains, “players who are actually using it for trading decisions are few and far between. You may have some of the more quantitative shops attempting to do that, because they have the staff. But not every asset manager or hedge fund has a team of data scientists.”
The report, based on responses from over 720 buy-side professionals, found that full adoption of AI and ML in the corporate bond market stood at just 21%, with an additional 34% reporting some adoption.
“Our studies found that the use of AI and ML was most widespread in municipal bonds … but we don’t necessarily believe that to be true. We think that could be due to a lack of understanding of what qualifies as AI/ML,” Blater tells WatersTechnology.
Nonetheless, an ongoing shift toward a more data-driven approach to markets means that the need for automation is greater than ever. A growing emphasis on best execution must be met with more deterministic methodologies. So if the penetration of advanced technologies is less advanced than the market thinks, it only means that the scope for disruption is greater.
Where we are now
As it stands, automation tends to be limited to specific, well-established use cases, mostly on the pre-trade side. Spencer Lee, chief markets officer and head of fixed income at TS Imagine, says greater adoption will come as awareness of the potential gains grows.
“The buy-side fixed-income community is trying to realize what is possible and achievable. Some players are undoubtedly utilizing AI and automation well, but the majority is still figuring it out,” says Lee, who spent five years at BlackRock and four years at hedge fund Agilon Capital before joining the vendor last year.
Operators of electronic trading platforms have been leading the charge when it comes to automation. With a constant flow of transactions worth billions of dollars a day, they have a rich stream of data to mine. This helps them to hone algorithms, impute prices, and train AIs.
Chris Bruner, chief product officer at Tradeweb, says that although developments in advanced technology are very promising, clients are not yet looking to chatbots for trading ideas and help with execution.
“Now that large language models (LLMs) are getting so much attention, there is a risk of automation being overhyped. But with that said, there’s a full spectrum of very practical applications that have been around for 15 to 20 years that are strongly impacting workflows today,” Bruner says.
One function that has developed significantly as a result of automation is price formation. Tradeweb’s bond-pricing engine AI-Price, for example, updates the prices of 30,000 securities every 30 seconds without relying on human interpretation to adjust curves. Regular pricing is particularly important in the fixed-income universe, which has a long tail of low-velocity instruments, making it hard for traders to value less liquid instruments.
This is fertile ground for ML, which is well suited to processing data from a range of categories that vary in firmness (in this case indicative prices, requests for quote, and traded prints). Models like this need to weigh up each data point as it comes in to determine how relevant it could be.
Take, for example, MarketAxess’ CP+. The ML-driven pricing model ingests behavioral data from trading as well as historical data from the company’s post-trade reporting business to generate the next bid, ask, buy, or sell for any bond. The model constantly assesses and hones its own accuracy by checking its predictions against the actual trading prices each day.
“One of the benefits of using ML is that you don’t have to have any specific data points or features related to the bond you want to trade. We can see whether or not similar bonds correlate in terms of their pricing behavior, and then use those to impute the price of the original bond,” says Gareth Coltman, global head of automation at MarketAxess.
Tradeweb’s Bruner adds that the iterative learning function is a key advantage of ML and AI versus other statistical learning approaches. “You get a feedback loop: You have all the data, clients execute, you see what cost they achieved on those executions, and then you keep turning the wheel. You learn more about what clients want to do,” Bruner says.
Automated functions are beginning to appear on the execution side, too. Algos and rules-based trading methodologies are gaining popularity. Apu Shah, partner at 28Stone Consulting, explains that the primary function of such technologies is to trade odd lots and smaller trades, freeing up space on the trading desk by reducing the need to gather information and make the necessary evaluations. “It’s really a question of ‘shove it in the algo [and] if the algo says it’s worth trading, trade it.’ That gives the human traders time to focus on the higher-value trades, the 5-million-and-up type of block trades,” Shah says.
MarketAxess’ Auto-X is one such tool. It allows traders to input parameters, such as the number of levels the machine must sample, the tolerance between the best price and the next best price, and the need for the best price to match or beat the reference price provided by CP+. If all the requirements are not met, the trader will be alerted so that they can take over and interact with the trade.
Last year, MarketAxess reported a record 380,000 trades completed using automated execution on Auto-X, a 58% increase on the year prior.
“People started out automating very small trades in very liquid bonds. As clients’ confidence in automation increased, we saw them gradually push the boundaries up to larger ticket sizes, and into less liquid bonds and markets where they weren’t traditionally using automation,” Coltman says. “For our biggest clients, on average around 40% of their trades are automated. But we do have some clients doing 80% or 90% of their activity that way.”
Where we’re headed
With interest in existing solutions seemingly on the rise, the final step in the process will be to use automation not just for smaller size, low-risk trades, but as a trade enhancement solution that can generate alpha in its own right.
The perennial problem of information leakage is high on the agenda. “That’s an area where we’re actively looking at the tools to improve. … If I’m only going to choose three, four, maybe five counterparties to send this request to, I really have to do a good job picking the counterparties I go to,” says Tradeweb’s Bruner.
Broadridge’s bond trading system subsidiary LTX provides a glimpse of what is to come in this respect. A neural network processes the history of a dealer’s trades along with the market conditions at the time and suggests the most likely natural counterparties for future deals. In the event that liquidity is hard to come by, it analytically suggests alternative bonds for which the system can see liquidity to reduce dependence on dealer capital.
For TS Imagine’s Lee, the future of automation is a “smart protocol chooser”. Where buy-side trading desks currently automate order engagement on one venue or protocol, he says, they will soon be able to put orders through an automated process that can scan multiple venues and protocols.
“We are moving toward giving the buy-side trading community the tools to build an algo that can look across all liquidity and protocol choices and create settings identifying the urgency of the trade or preferencing a protocol based on information leakage. These are just some examples in a decision tree that could be automated without any handholding,” Lee says.
In the meantime, innovation is receiving a welcome tailwind from fundamental changes in the approach to trading. The rise of a more data-driven methodologies in portfolio management and execution is a boon for fixed-income markets, where causality and correlation should become easier to identify in datasets.
“Deterministic behavior is much riper for automation. It’s quite easy to automate a process where you can see ‘if this is the set of inputs, then my decision will be this,’” says Coltman.
Once you’ve coded something into the computer, it’s going to do what it was instructed to do. But these trades often require a trader to adjust and try again if one element doesn’t go to plan.
Apu Shah, 28Stone Consulting
Another boost could come from the import of technologies from other financial markets where automation is more developed. Roger Burkhardt, CTO for capital markets and AI at Broadridge, has experience in the equities world from his time as CTO of NYSE during the move to electronic execution. He can see parallels between past shifts in the equities market and what is currently happening in the world of fixed income.
“In the equities space, the number of sales traders at any one institution has shrunk significantly,” Burkhardt says.
“Now it is trader technologists who are creating rules and building algos. I think the fixed-income market is accelerating down a similar path to greater automation, and the technologies that have been around for a number of years in the equities world are migrating into the fixed-income world. It’s really an innovation diffusion.”
Full automation of fixed-income trading remains unlikely, though. Complex, multi-strategy trades are hard to program into a computer. “There’s also the question of pivoting,” Shah says. “Once you’ve coded something into the computer, it’s going to do what it was instructed to do. But these trades often require a trader to adjust and try again if one element doesn’t go to plan.”
Coltman agrees that there are still a lot of high-touch trades that will always need to be managed by a trader. “But it’s important to recognize that trader discretion sometimes leads to bias. Over time, biases can yield outcomes that are not necessarily the best in terms of execution performance, so the right balance between trader and machine needs to be struck.”
‘Fear of the machine’ and other obstacles
The spread of automation is held back by some uniquely human problems, though.
“I think we still have quite a long way to go when it comes to automation. One reason for that is a fear of the machine,” says Shah.
He says that during the last algo trading project that he worked on, his team spent around 60% of the implementation time on risk controls. “‘How do we make sure that this algo never goes rogue? What if my internet is down and I can’t click that circuit breaker? Can we make it so that I can text a phone number and it automatically stops?’ These types of fears play into how much firms are willing to give up those high-value trades. There’s not enough confidence yet,” he says.
TS Imagine’s Lee agrees. “In my experience, much of the buy-side fixed-income community is cautious about the potential dangers of automation. When you turn the machines on, you just let them run, but the consequences of mistakes or failings can be very high,” Lee says.
The idea of automation going off the rails dredges up harrowing memories of the 2012 incident when rogue software at trading firm Knight Capital booked the company $440 million in losses in a day and wreaked havoc on markets.
This may have been a factor in the Securities and Exchange Commission’s (SEC’s) recent announcement that it would look into the way brokers use AI. But while emerging technologies can be daunting, experts say the root cause of such meltdowns is usually the underlying data and not the technology itself.
“Who’s to say that the addition of AI or ML is any riskier than rules-based algorithms?” Coalition Greenwich’s Blater asks. “Currently, if somebody feels like saying, ‘if the market moves 5%, you keep going,’ they can theoretically do that. And that’s a huge market move in equities. But in bonds, it can happen when someone sneezes, depending on liquidity.”
Another difficulty lies in the explainability of automated processes. It can be difficult to understand precisely why a machine has come to a given conclusion, which makes it harder to demonstrate best execution and to explain to portfolio managers what their options are.
Eugene Grinberg, co-founder and CEO of market data platform Solve, says the explainability problem risks tempering the enthusiasm for automation. “Having spoken to some people in the market, people are excited, but they’re very aware that it’s a black box. At the end of the day, you have no idea what are the coefficients that the model has learned based on somebody’s historic relationship, and what kind of results it’s going to produce in some sort of edge case,” Grinberg says.
The data is there. The skill sets are there. It’s definitely happening now.
Chris Bruner, Tradeweb
Still, proponents of automation believe the market will overcome its wariness once it sees the results that automation can yield. “For us, the KPIs and the objective functions are probably even more important than the explainability. Once a technology has been used for thousands of trades, you can show people the data and demonstrate that KPIs and objective functions are improving through time,” says Tradeweb’s Bruner.
The final—and most intractable—obstacle to greater automation, though, is market structure, as fixed income is heavily fragmented. A significant portion of securities across the fixed-income ecosystem are still traded by phone and email, and those that are traded electronically are divided between a growing number of liquidity pools.
Solve has access to clients’ emails in which pricing information is exchanged, and digitizes bids and offers using natural language processing (NLP). It then uses ML to clean up the data and extract 20 million quotes per day across different asset classes.
But the difficulty of identifying and comparing bonds remains significant. “The fixed-income ecosystem is just so vast, and bonds are so unique that identifying securities that are true comparables is not trivial,” Grinberg says.
He continues: “We have been working on converting all the many millions of data points that we collect into some sort of a coherent yield surface—market summaries where we’re able to identify historical trends around specific cohorts in the market. That would allow you to identify outliers between individual securities relative to their cohort. That’s the next phase: converting data into information.”
Granted, we may be early on in the adoption curve. But given the complexity of the fixed-income market, the fact that technologists are discussing how best to employ automated processes using the data they have already collected and cleaned is no small achievement.
“Clients used to talk about how they would get the data, and then maybe how they would use the data and which systems they would use to ingest, normalize, and present it,” says Tradeweb’s Bruner. “I think they’ve moved past that stage, and now they’re focused on how to use the data to improve and automate their process. So the data is there. The skill sets are there. It’s definitely happening now.”
Only users who have a paid subscription or are part of a corporate subscription are able to print or copy content.
To access these options, along with all other subscription benefits, please contact info@waterstechnology.com or view our subscription options here: http://subscriptions.waterstechnology.com/subscribe
You are currently unable to print this content. Please contact info@waterstechnology.com to find out more.
You are currently unable to copy this content. Please contact info@waterstechnology.com to find out more.
Copyright Infopro Digital Limited. All rights reserved.
You may share this content using our article tools. Printing this content is for the sole use of the Authorised User (named subscriber), as outlined in our terms and conditions - https://www.infopro-insight.com/terms-conditions/insight-subscriptions/
If you would like to purchase additional rights please email info@waterstechnology.com
Copyright Infopro Digital Limited. All rights reserved.
You may share this content using our article tools. Copying this content is for the sole use of the Authorised User (named subscriber), as outlined in our terms and conditions - https://www.infopro-insight.com/terms-conditions/insight-subscriptions/
If you would like to purchase additional rights please email info@waterstechnology.com
More on Emerging Technologies
Quants look to language models to predict market impact
Oxford-Man Institute says LLM-type engine that ‘reads’ order-book messages could help improve execution
The IMD Wrap: Talkin’ ’bout my generation
As a Gen-Xer, Max tells GenAI to get off his lawn—after it's mowed it, watered it and trimmed the shrubs so he can sit back and enjoy it.
This Week: Delta Capita/SSimple, BNY Mellon, DTCC, Broadridge, and more
A summary of the latest financial technology news.
Waters Wavelength Podcast: The issue with corporate actions
Yogita Mehta from SIX joins to discuss the biggest challenges firms face when dealing with corporate actions.
JP Morgan pulls plug on deep learning model for FX algos
The bank has turned to less complex models that are easier to explain to clients.
LSEG-Microsoft products on track for 2024 release
The exchange’s to-do list includes embedding its data, analytics, and workflows in the Microsoft Teams and productivity suite.
Data catalog competition heats up as spending cools
Data catalogs represent a big step toward a shopping experience in the style of Amazon.com or iTunes for market data management and procurement. Here, we take a look at the key players in this space, old and new.
Harnessing generative AI to address security settlement challenges
A new paper from IBM researchers explores settlement challenges and looks at how generative AI can, among other things, identify the underlying cause of an issue and rectify the errors.