The push for AI to unlock elusive automation in bond trading
Calculating total market capacity is key to automating the end-to-end execution of large bond trades, says Vuk Magdelinic.
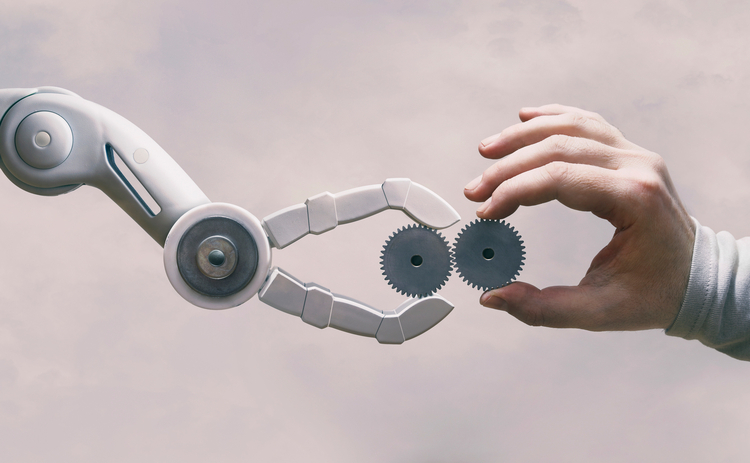
Imagine you’re a tech-themed, bond fund trader and need to rebalance the fund immediately. To do so, you need to buy $50 million of a specific 10-year bond of a major telecommunications company. However, the largest amount of this bond traded on any day in the last six months is only $2 million. What do you do?
Traders usually execute large bond trades like this over the phone, with buy-side traders relying on a broker-dealer’s capability to source bonds to fill the trade. Some market observers argue that the need for dealer services in these situations will prevent the full adoption of electronic trading protocols. But advances in the application of technology and data science are solving the problem of end-to-end automated bond trading for any ticket size.
The traditional assumption is that the client will get preferential treatment as trade size increases, and the bid–ask spread at which the client can execute will be tighter than for a smaller trade. But, on average, there’s only $250 million of a bond outstanding, and only a portion of that trades daily. So, as the size of a bond trade increases, a point is reached where there’s not enough of the bond readily available to fill the trade. Beyond this point, the bid–ask spread begins to widen as the dealer must add the cost of their liquidity discovery services to the trade. These costs reflect the extra work the trader must do to find holders of the bond and the potential premium the trader must pay to these sellers to convince them to sell the bond.
Systematically determining total market capacity for any bond issue is crucial for automated bond trading, but no one has been able to model TMC properly
Vuk Magdelinic, Overbond
This point is the bond’s total market capacity (TMC). It represents the portion of the outstanding amount of the bond not tied up by buy-and-hold accounts that are unwilling to sell. Systematically determining TMC for any bond issue is crucial for automated bond trading, but no one has been able to model TMC properly.
Aggregating the right data is one of the main hurdles to modeling TMC. No centralized, real-time bond trade datafeed with trade sizes attached to trade prices exists. To get around this, post-trade transaction data, which includes size information, is used for modeling TMC. Unfortunately, post-trade data is, by definition, delayed and often size-masked for trades beyond a certain threshold.
Here, the power of AI makes previously impossible analysis possible. AI learning and prediction are applied to six or more months of historical data to bridge the delay and discern trade size and volume patterns. For instance, by tracking what is added daily, the algorithm uses Trace data to determine how much inventory dealers are adding incrementally over several months.
Automated trading needs TMC
Knowing the TMC is valuable information for traders trying to determine prices for voice or manual electronic trades. When incorporated into automated trading, it makes pricing more precise, smart order routing (SOR) more efficient and margin calculations applicable to a larger range of trades.
SOR is an automated process used by the buy side for online trading. It executes trades by allocating them across various electronic trading venues and counterparties based on price and liquidity to get the best execution and minimize training costs. Before allocating a trade, an SOR algorithm must determine how to chunk the entire trade size into pieces—a process that will be less efficient if TMC is unknown.
TMC is also necessary for automating sell-side trading. Broker-dealers, competing to win trades in a request-for-quote (RFQ) protocol, try to minimize their margin, which is the distance of their quote from the next-best quote by a competitor (which market etiquette entitles them to know). Traders try to optimize margin to be more profitable, and they’re likely to alter it dramatically as they trade past the TMC. An automated AI margin optimization algorithm must reflect this to be effective.
Thankfully, using data science and AI has solved the problem of incorporating TMC into both buy-side and sell-side automated bond trading. Now, a buy-side trader can rely on end-to-end automated trading to execute a ticket of any size and sell-side traders can profitably respond to RFQs of any size.
Vuk Magdelinic is the founder and CEO of Overbond, which provides quantitative analytics for the institutional fixed income space
Only users who have a paid subscription or are part of a corporate subscription are able to print or copy content.
To access these options, along with all other subscription benefits, please contact info@waterstechnology.com or view our subscription options here: http://subscriptions.waterstechnology.com/subscribe
You are currently unable to print this content. Please contact info@waterstechnology.com to find out more.
You are currently unable to copy this content. Please contact info@waterstechnology.com to find out more.
Copyright Infopro Digital Limited. All rights reserved.
You may share this content using our article tools. Printing this content is for the sole use of the Authorised User (named subscriber), as outlined in our terms and conditions - https://www.infopro-insight.com/terms-conditions/insight-subscriptions/
If you would like to purchase additional rights please email info@waterstechnology.com
Copyright Infopro Digital Limited. All rights reserved.
You may share this content using our article tools. Copying this content is for the sole use of the Authorised User (named subscriber), as outlined in our terms and conditions - https://www.infopro-insight.com/terms-conditions/insight-subscriptions/
If you would like to purchase additional rights please email info@waterstechnology.com
More on Data Management
The IMD Wrap: Talkin’ ’bout my generation
As a Gen-Xer, Max tells GenAI to get off his lawn—after it's mowed it, watered it and trimmed the shrubs so he can sit back and enjoy it.
Waters Wavelength Podcast: The issue with corporate actions
Yogita Mehta from SIX joins to discuss the biggest challenges firms face when dealing with corporate actions.
Data catalog competition heats up as spending cools
Data catalogs represent a big step toward a shopping experience in the style of Amazon.com or iTunes for market data management and procurement. Here, we take a look at the key players in this space, old and new.
This Week: JP Morgan, Broadridge, Lloyds, JSE, Schroders, and more
A summary of the latest financial technology news.
What firms should know ahead of the DSB’s UPI launch
Six jurisdictions have set deadlines for firms to implement the derivatives identifier, with more expected to follow.
Has cloud cracked the multicast ‘holy grail’ for exchanges?
An examination of how exchanges—already migrating to the cloud—are working to solve the problem of multicasting in a new environment.
Waters Wrap: Market data spend and nice-to-have vs. need-to-have decisions
Cost is not the top factor driving the decision to switch data providers. Anthony looks at what’s behind the evolution of spending priorities.
The consolidated tapes are taking shape—but what shape exactly?
With political appetite established on both sides of the Channel, attention is turning to the technical details.